LiDAR Design Lab: Safeguarding AI
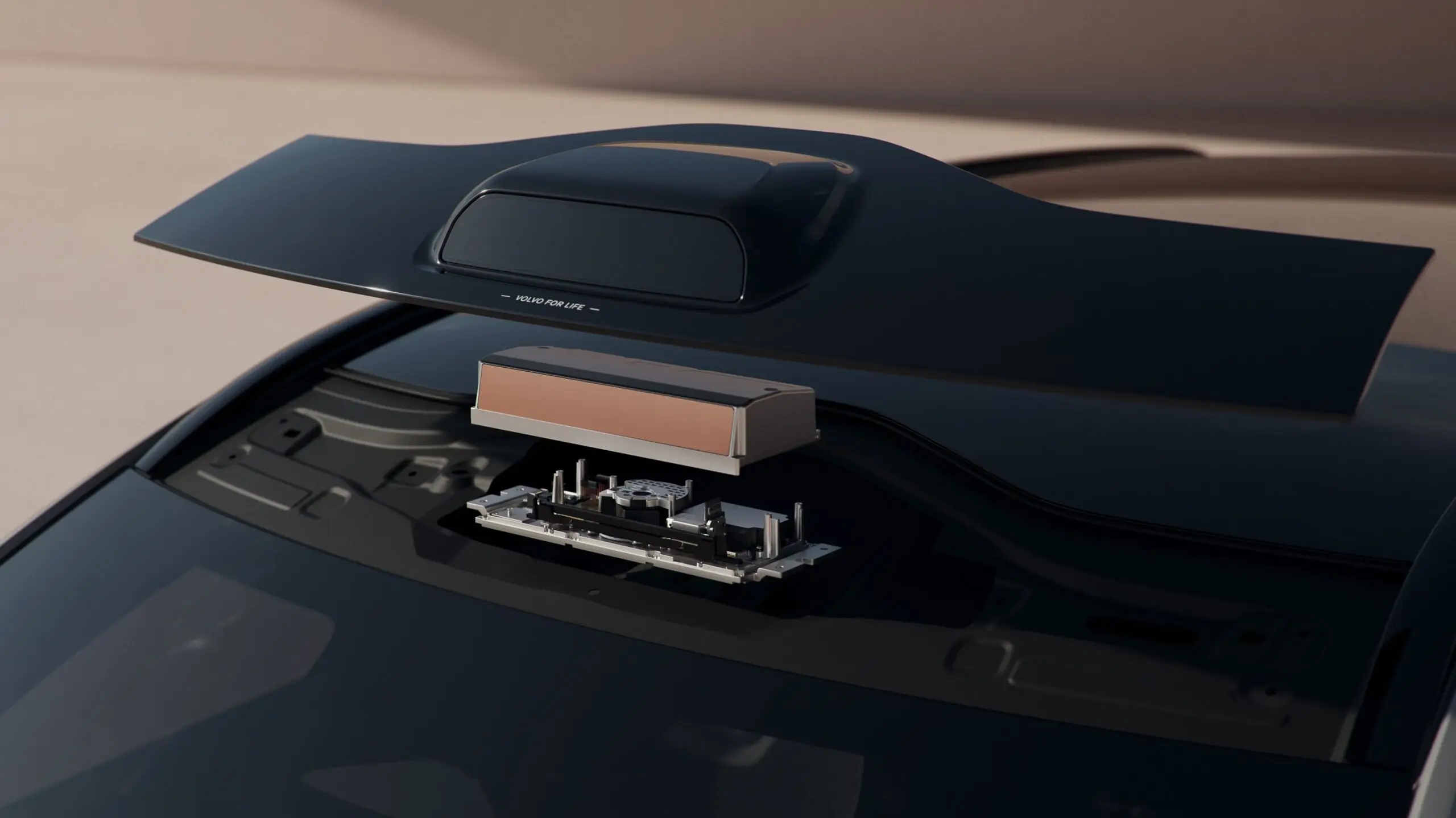
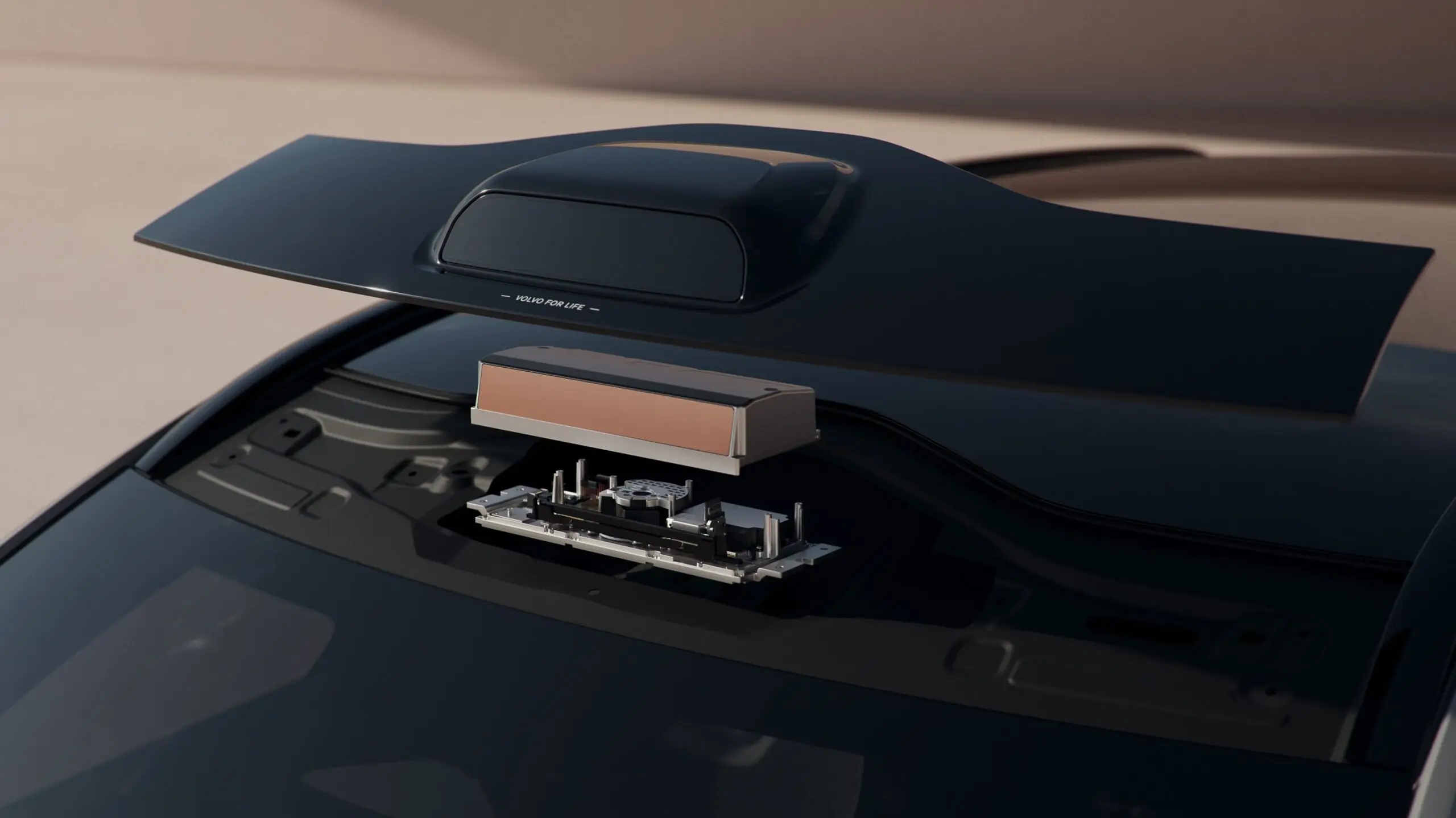
Deterministic safeguards can enable both emerging and established AI systems to address safety-critical applications.
AI models famously exhibit unknown points of failure (commonly called AI hallucinations) because of a fundamentally inference-based output and a reliance on training data breadth and depth. Automotive AI systems suffer from both hallucinations and the inability to see objects and obstacles they have not been trained to recognize.
Burden of Training: Training is both nuanced and expensive. AI brings forward biases and inaccuracies of the dataset used to train it (e.g. facial recognition). These biases are difficult to predict beforehand, and in automotive applications can have safety-critical consequences.
Endless Edge Cases: Even when a training methodology is thorough, there are still unknown points of failure when the trained system experiences scenarios sufficiently different from its training set. General obstacles like road debris, for example, can have such a diverse appearance that training a system to recognize them on sight may require prohibitively large amounts of training data.
AI System Enablement: A deterministic safeguard provides a cost-effective way to deploy emerging AI systems and mitigate operational risk for both emergent and established systems by serving as the basis for an Automotive Safety Integrity Level (ASIL) and Safety of the Intended Functionality (SOTIF) safety rating. This lets the AI systems lean forward and deploy with real risk of aberrant AI inferences (i.e. the bias-variance tradeoff).
LiDAR is the only sensor type that provides this deterministic 3D measurement at sufficient range & resolution.
The inherent information captured by LiDAR is three dimensional, as is the environment the vehicle needs to navigate.It is therefore possible to generate a distillation of that 3D environment that can act as a provable set of boundaries – free of probability, training, and inference. This is unique to LiDAR because, unlike camera and radar, the LiDAR can tell you where there is nothing (drivable freespace) in addition to where there are obstacles.
Luminar LiDAR delivers the longest-range freespace detection as well as the most accurate obstacle size estimation.
Luminar’s LiDAR architecture (1550nm, narrow divergence, linear time of flight) enables detection of the hardest targets with high fidelity. This means the vehicle can directly detect and understand the nuance of what space is readily drivable (road), cautiously drivable (gravel, grass), and not drivable (sidewalk).
Luminar’s Sentinel: With all this in mind, a central theme of Luminar’s software in development is a dual-path approach running sensor data through both a purely classical pipeline (the safeguard) and an AI pipeline (the classifier & predictor). This allows us to deliver the best of both algorithmic worlds and enable the safest and most performant vehicle systems in the world.